Table of Contents
In the fast-expanding field of machine learning, having the correct server may significantly enhance project productivity and results. Choosing the finest server for machine learning is best for both startups and large enterprises.
This post will help you choose the best server for your machine learning needs in 2024 by concentrating on four essential factors: GPU performance, CPU performance, RAM and storage, and scalability and expansion.
How to Choose a Server for Machine Learning
When it comes to machine learning, the server you choose can greatly influence the speed and efficiency of your computations. Given the intensive nature of machine learning tasks, selecting a server that meets your requirements is crucial. Let’s break down the critical components to consider:
Contact us
1. GPU Performance
The GPU is the heart of machine learning operations, accelerating the computation process significantly. When looking for the best server GPU, consider the NVIDIA range, which is renowned for its robust performance in machine learning applications. The GPU server price can vary, so balancing cost and performance is essential based on your specific needs.
2. CPU Performance
While GPUs handle the majority of the computational load in machine learning projects, the CPU’s importance cannot be underestimated. It handles additional processes that the GPU isn’t doing, making it an essential component of your server. The Intel Xeon CPU list includes a variety of suitable solutions for server environments, ensuring that your machine-learning operations function smoothly.
3. Memory and Storage
Memory and storage are crucial for machine learning servers as they affect data retrieval speeds and the volume of data that can be processed simultaneously. Opt for servers with high RAM capacities and fast, reliable storage solutions to facilitate efficient data processing and storage.
4. Scalability and Expansion
Your machine learning needs might grow or change, requiring more resources. Therefore, choosing a server that offers scalability and expansion capabilities is wise. This ensures that you can upgrade your hardware as needed without investing in a new server entirely.
Performance Level: How to Compare GPUs for Machine Learning
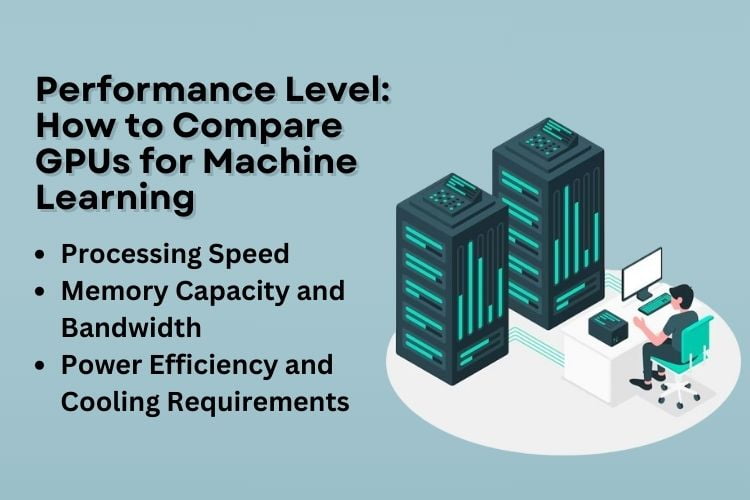
Choosing the correct GPU is critical for machine learning applications. It can have a major impact on your model’s training duration and efficiency. To help you make an informed selection, we’ll go over how to evaluate GPUs for machine learning, concentrating on processor speed, memory capacity, and bandwidth, as well as power efficiency and cooling needs.
1. Processing Speed
When comparing GPUs for machine learning, processing speed is a key factor to consider. It determines how fast your machine learning models can be trained and how quickly they can process data.
Higher processing speeds mean shorter wait times for your models to learn and adapt, making your machine-learning tasks more efficient. Look for GPUs known for their robust processing capabilities, especially those desirable in a machine-learning community.
2. Memory Capacity and Bandwidth
Memory capacity is crucial as it dictates how much data your GPU can handle at any given time. For machine learning tasks, which often require handling large datasets, having a GPU with ample memory is beneficial.
Alongside memory capacity, high memory bandwidth is vital as it influences the speed at which data can be read from and written to the GPU memory. Higher bandwidth means your GPU can process more data simultaneously, enhancing its overall performance.
3. Power Efficiency and Cooling Requirements
Power efficiency is another critical aspect to consider when comparing GPUs for machine learning. Efficient power usage not only reduces operational costs but also lessens the environmental impact. Moreover, GPUs that consume less power typically generate less heat, which brings us to another important consideration: cooling requirements.
Adequate cooling is essential to maintain optimal performance and prevent overheating, which can lead to hardware failure or throttled performance. Thus, assessing a GPU’s power efficiency and cooling needs is essential for maintaining a sustainable and reliable machine-learning environment.
Price vs. Performance: Choosing the Best Value for Machine Learning GPU
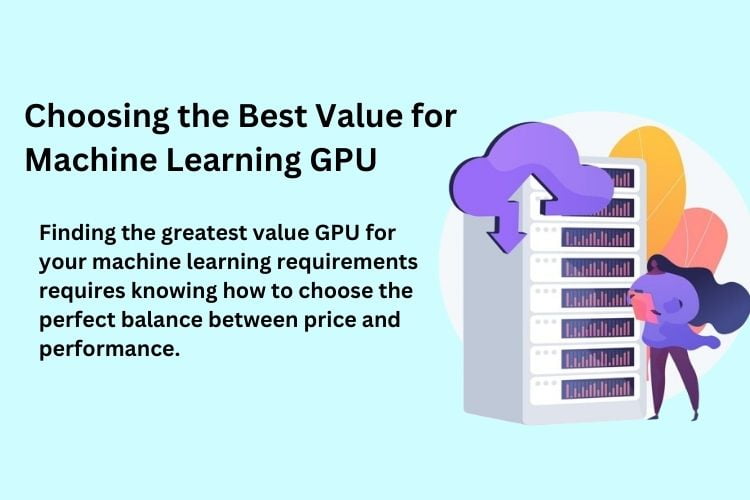
Choosing the right GPU is essential when exploring machine learning since it affects both performance and cost. Finding the greatest value GPU for your machine learning requirements requires knowing how to choose the perfect balance between price and performance.
1. Budget Options with High Performance-to-Cost Ratios
For individuals and organizations conscious of their spending, there are budget-friendly GPUs that offer high performance-to-cost ratios. These GPUs provide a cost-effective solution for machine learning projects without significantly compromising on performance.
By choosing a budget-friendly option, you can still enjoy robust machine learning capabilities, making these GPUs ideal for those starting or those with limited financial resources.
2. Premium Options for Uncompromised Performance
For those seeking the pinnacle of machine learning performance and who are less constrained by budget, premium GPUs offer top-tier performance. These units are designed for demanding tasks and can handle complex machine-learning algorithms with ease.
While they come at a higher price point, their performance benefits can be crucial for advanced projects and applications where time and accuracy are of the essence.
Real-World Application Case Studies for GPUs in Machine Learning
GPUs have been instrumental in advancing various fields through machine learning. Below are real-world applications where GPUs have significantly contributed to progress and innovation.
1. Image and Speech Recognition
GPUs are speeding up progress in image and speech recognition by allowing for faster data processing and more complicated calculations. Large datasets must be analyzed for these applications, and GPUs speed up processing to get faster, more accurate results.
2. Predictive Analytics and Data Mining
Predictive analytics and data mining are critical for businesses and researchers, helping predict trends and outcomes. GPUs have empowered these fields by handling large volumes of data more efficiently, thereby enhancing the precision and speed of predictive models.
3. Natural Language Processing and Translation
Natural language processing and translation benefit immensely from GPUs, as they require analyzing and processing vast amounts of textual data. GPUs accelerate these tasks, enabling more nuanced and contextually accurate language models.
Read More:- How to choose the best server for large businesses in 2024 in India
Challenges and Factors to Consider When Selecting a GPU for Machine Learning
Selecting the right GPU involves navigating various challenges and considerations to ensure it aligns with your project’s needs.
Compatibility and Integration Challenges
Ensuring that your chosen GPU is compatible with your existing systems and software is crucial. Compatibility issues can lead to underutilization of the GPU’s capabilities or, worse, prevent it from working with your machine learning environment.
Scalability for Larger Projects
Considering the scalability of your GPU solution is vital, especially for growing or evolving machine learning projects. The GPU should be able to handle increased workloads and data sizes without bottlenecking performance.
Energy Consumption and Cooling Needs
GPUs can be energy-intensive, and high-performance models may require significant cooling. Balancing energy consumption with cooling requirements is essential to maintain efficiency and prevent overheating, especially in budget-friendly GPU options where these factors can vary widely.
By carefully evaluating these aspects across different GPU options, you can make an informed decision that balances price, performance, and practicality for your machine learning projects.
Conclusion
Combining cost and performance is crucial when searching for the best hardware for your machine-learning initiatives. The secret is to select a device that fits the scope and complexity of your project, whether you’re buying a GPU for faster data processing or going with a full-featured server solution.
Serverstack proves to be a reliable partner by providing a variety of server alternatives to suit different goals and budgets. You’re investing in a foundation that supports the expansion and success of your machine-learning activities by selecting a Serverstack server, which guarantees that your computing resources are reliable, scalable, and reasonably priced.
Frequently Asked Questions
Q1. What is a server in machine learning?
In machine learning, a server refers to a powerful computer that provides the necessary computational resources to train and run machine learning models. It typically has high-performance CPUs, GPUs, ample memory, and significant storage capacity to handle large datasets and complex computations.
Q2. What is the best GPU for machine learning in 2024?
The best GPU for machine learning in 2024 would likely be one that offers outstanding performance, extensive memory, and efficient processing capabilities. While specific models can vary, GPUs from NVIDIA’s latest series are often considered top-tier for machine learning due to their advanced architecture and high processing power.
Q3. Why is GPU faster than CPU for machine learning?
GPUs are better than CPUs in machine learning due to their high number of cores built for parallel computing, which is best for the matrix and vector operations found in machine learning. This enables GPUs to perform several operations simultaneously, dramatically speeding up the training and execution of machine learning models.
Q4. Which machine-learning GPU server is best?
Choosing the right machine-learning GPU server, like the ASUS ESC 4000 G2S, depends on what you need. If you have big projects, a limited budget, and need high performance, servers with top-notch NVIDIA GPUs are usually the best. They’re super powerful and work well with lots of different machine-learning tools.
Q5. What is the most efficient GPU for machine learning?
The most efficient GPU for machine learning is one that offers a balance between power consumption and computational capability. Efficiency can vary based on the workload and specific tasks. NVIDIA’s GPUs, particularly those designed with energy efficiency in mind, are often regarded as efficient choices for sustained machine learning workloads.
Q6. What is the minimum GPU required for machine learning?
The minimum GPU required for machine learning should be capable of supporting the specific frameworks and libraries you plan to use, such as TensorFlow or PyTorch. Entry-level GPUs from NVIDIA, like the GTX series, can be sufficient for basic or educational purposes. However, for more complex or data-intensive tasks, a more powerful GPU, such as those from the NVIDIA RTX series, would be recommended to ensure adequate performance and speed.